Broader Impact Highlights
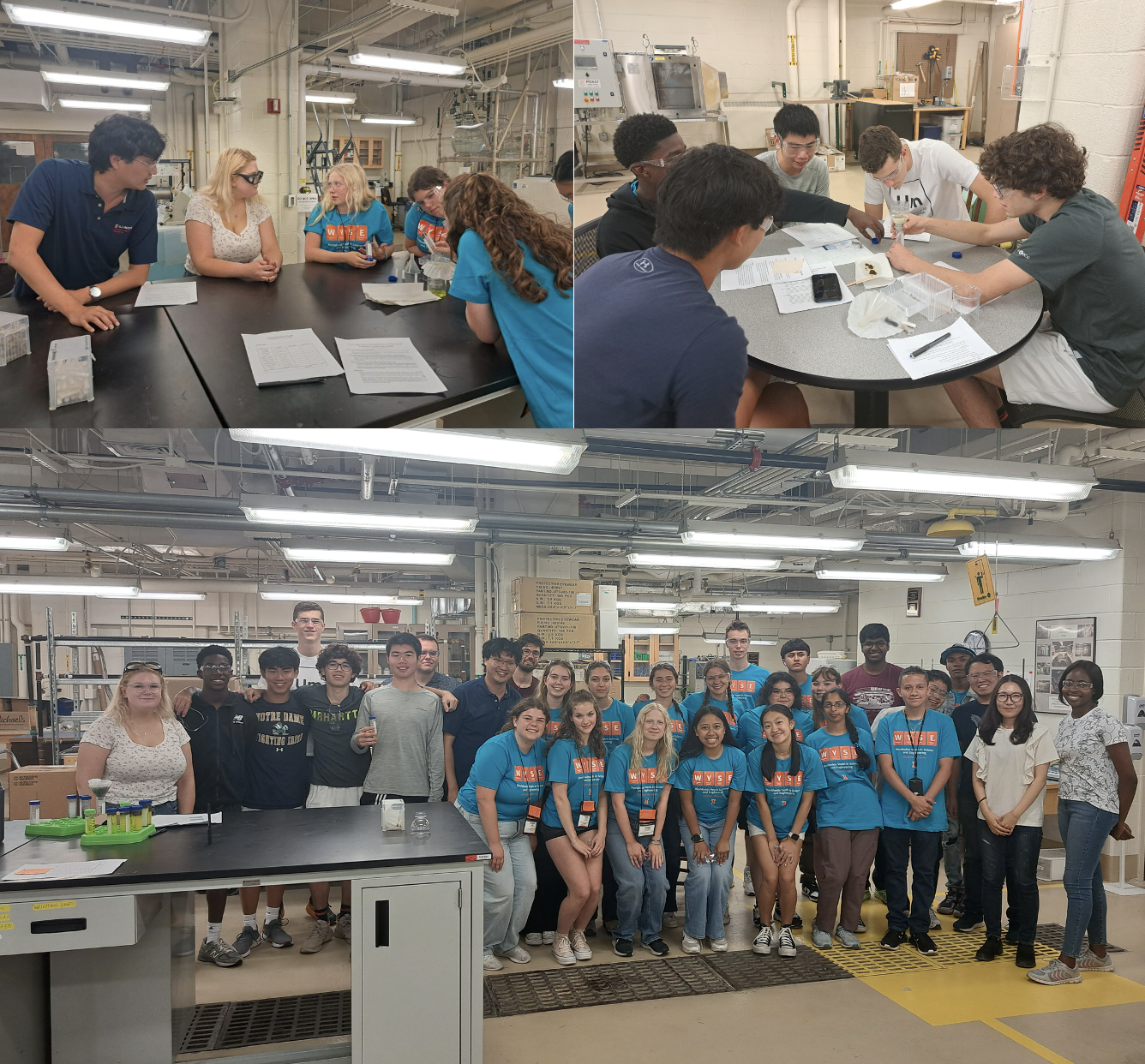
Chemical Engineering Summer Camp: Separation Science & Water Filtration
11/21/2024 | Xiao Su (University of Illinois Urbana-Champaign)
For undergrad and graduate chemical engineering education, Su and Calabrese taught Mass Transfer and Separations courses at UIUC (ChBE 422) and UMN (ChEn3006), providing a unique opportunity to train future engineers on emerging separations technologies.
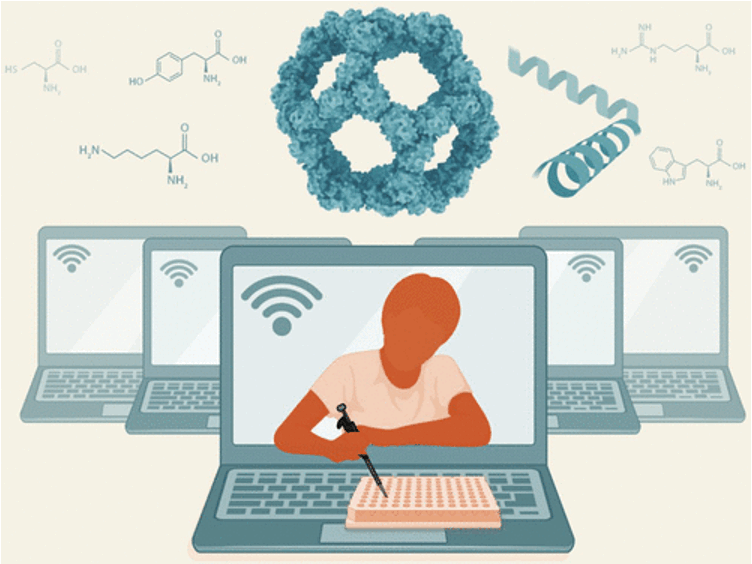
Increasing Computational Protein Design Literacy through Cohort-based Learning for Undergraduate Students
10/25/2024 | David Baker and Neil King (U. Washington)
This program provides a model of structured computational research training opportunities for undergraduate researchers in any field for organizations looking to expand educational access.
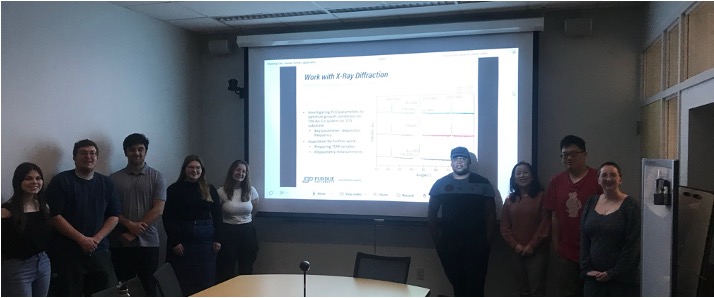
Undergraduate Research Involving Pulsed Layer Deposition (PDL) and Transmission Electron Microscopy (TEM)
6/24/2024 | Haiyan Wang (Purdue University)
This project supports 4 PIs and their graduate students in working on the collaborative project covering thin film growth, characterization, materials modeling, device simulation and processing
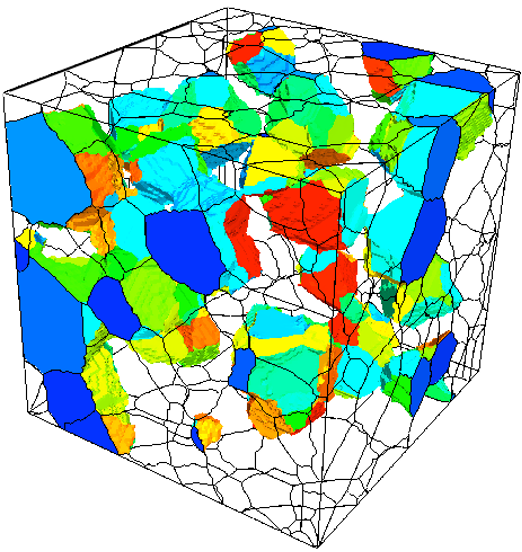
Broader Impact Through the 3D Microstructure Workshop
5/22/2024 | Gregory S. Rohrer, Carnegie Mellon University
The DMREF sponsored a three-dimensional (3D) microstructure workshop August 18-20, 2023. This was our most successful workshop so far, in the sense that we exceeded our limit for the maximum number of participants (60).
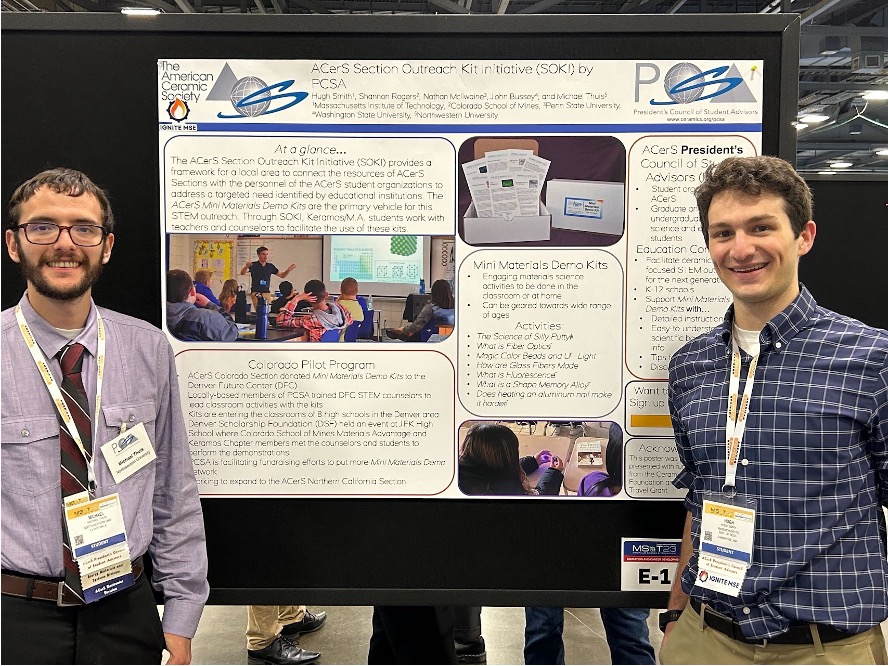
Distributing Materials Science Classroom Kits for High School Students
5/17/2024 | Mo, Hu, Haile, Harmer, Marvel Univ. of Maryland, Northwestern Univ., Lehigh Univ.
The outcome of the computation, experiments, and iterative feedback loop from this project are integrated into multiple courses taught by the PIs. This integration of research and education enriches the educational experiences and outcomes for students, fostering a new generation of scientists and engineers equipped with advanced MGI skills in computational and experimental materials science.
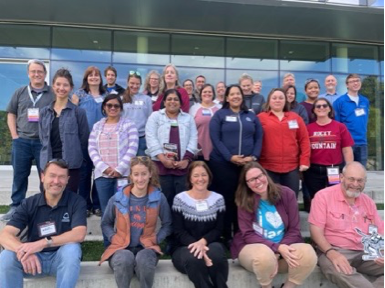
Crystals and Clinker Teacher Workshop
5/14/2024 | Geoff Brennecka (Colorado School of Mines)
Geoff Brennecka and the Ceramics and Glass Industry Foundation hosted the Crystals and Clinker Teacher Workshop at Colorado School of Mines on October 14, 2023, that introduced twenty regional middle- and high-school teachers to materials science concepts and activities.
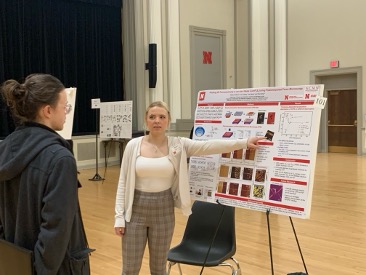
Undergraduate Research on 2D Ferroelectrics
5/14/2024 | Xia Hong (University of Nebraska-Lincoln) Li Yang (Washington University in St. Louis)
Layered van der Waals CuInP2S6 (CIPS) is a promising two-dimensional (2D) ferroelectric for developing low-power 2D electronics and flexible energy applications. A major hurdle for its technological application is its close to room temperature ferroelectric ordering temperature (TC). Our study provides an effective strategy to enhance the ferroelectricity and piezoelectricity of ultrathin CIPS, making it a viable material candidate for room temperature device operation. The gained knowledge can also be applied to other 2D ferroic materials for property engineering.
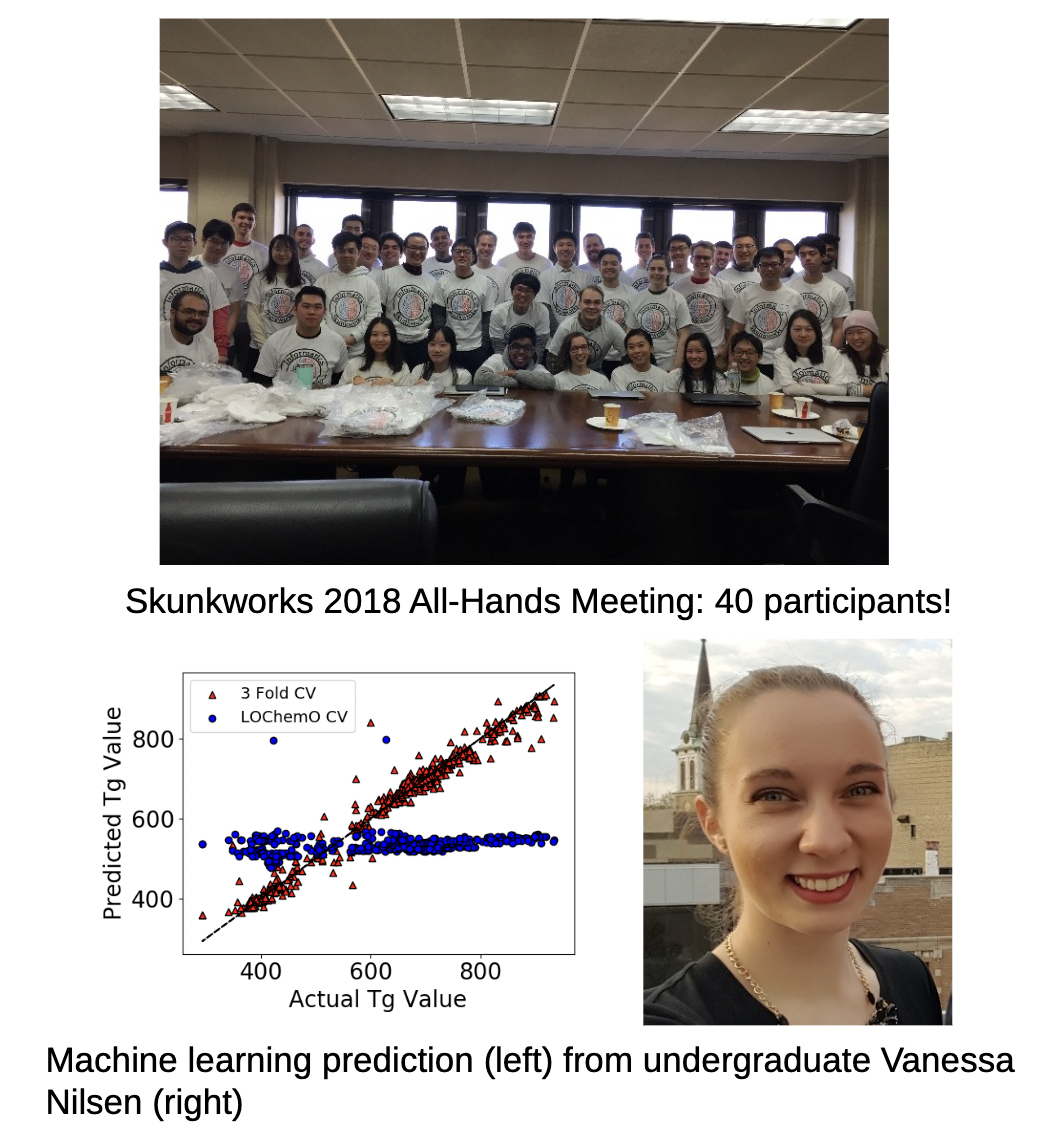
The Information Skunkworks
3/21/2023 | Dane Morgan and Paul Voyles (University of Wisconsin)
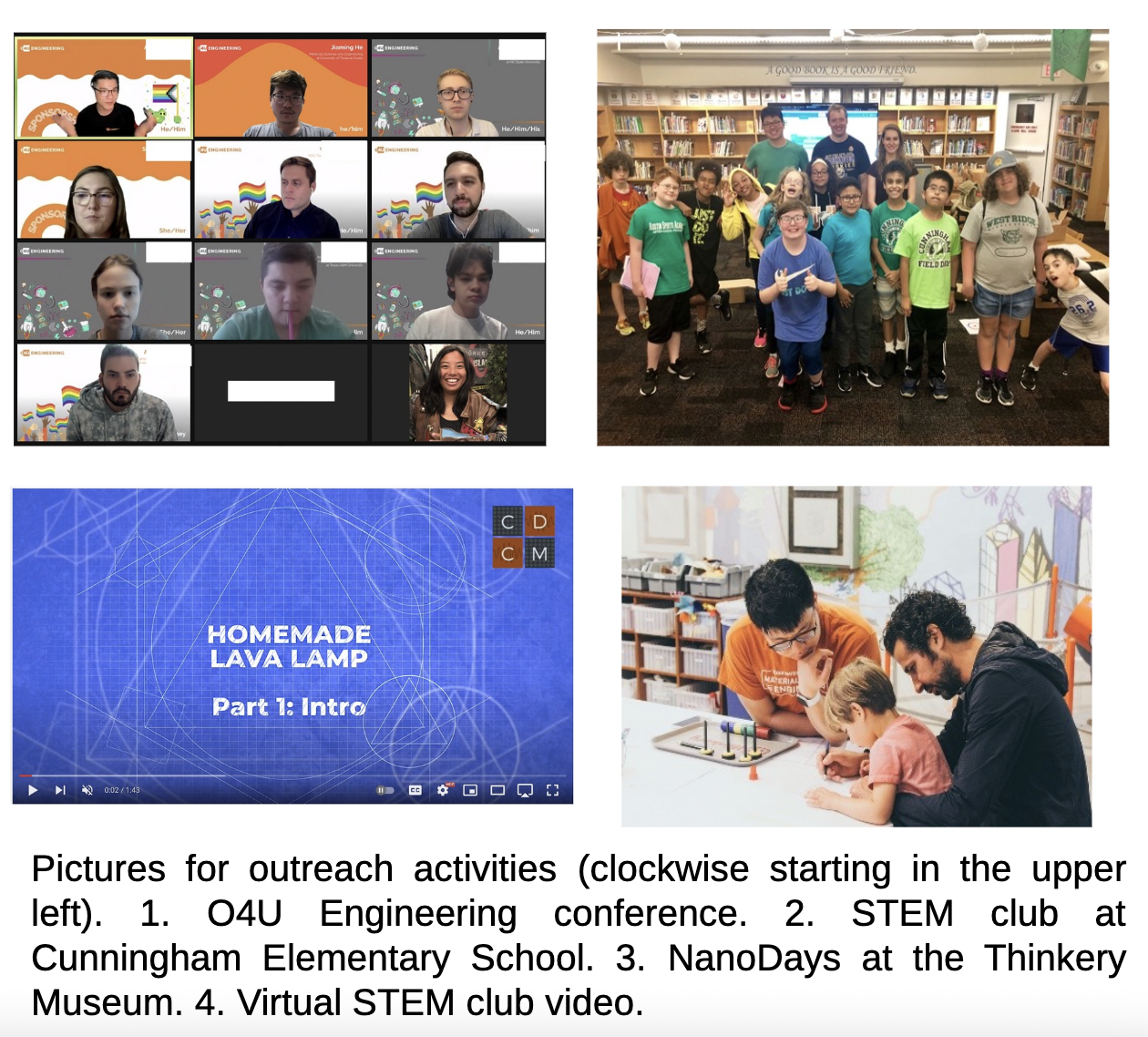
Evidence for Spin Swapping in an Antiferromagnet
3/2/2023 | J. Zhou (U. Texas-Austin); G. Fiete (Northeastern U.); C.L. Chien (Johns Hopkins U.)
In outreach activities, we have sought to inspire the next generation of researchers from different age groups and backgrounds. We have focused on building approachable and adaptive demonstrations of scientific experiments for K-12 classrooms, including in-person and virtual projects. We are also working with undergraduate students within the department on their research projects to kickstart their career.
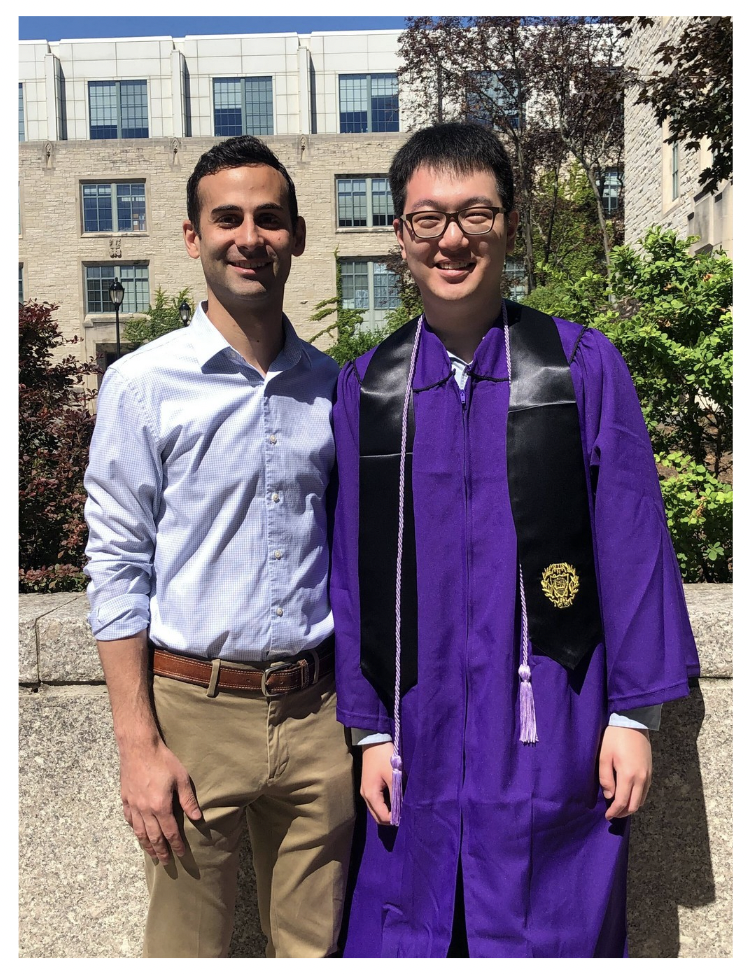
Workforce Development by Advancing MGI Competencies
2/21/2023 | James Rondinelli (Northwestern University)
The DMREF project advanced next generation workforce competencies in data handling, atomistic methods, advanced experimentation, and integrated workflows through data-driven research projects.
Showing 1 to 10 of 54