A Neural Network Approach for Catalysis
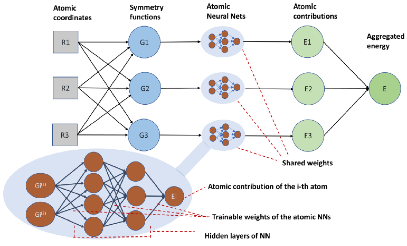
Computational catalyst discovery involves the development of microkinetic reactor models based on estimated parameters determined from density functional theory (DFT). For complex surface chemistries, the number of reaction intermediates can be very large, and the cost of calculating the adsorption energies by DFT for all surface intermediates, even for one active site model, can become prohibitive. Here, a detailed investigation has been performed on a predictive model for both interpolation and extrapolation of adsorption energies of hydrocarbon species on a Pt(111) catalyst surface. Appropriate descriptors and machine learning models have been identified that can predict a significant part of these adsorption energies given data on the rest of them. Thus, a neural network predictive model has been developed that combines an established additive atomic contribution-based model with the concepts of a convolutional neural network that, when extrapolating, achieves a statistically significant improvement over the previous models.