Targeted Materials Discovery using Bayesian Algorithm Execution
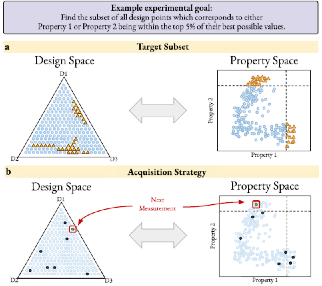
Rapid discovery and synthesis of future materials requires intelligent data acquisition strategies to navigate large design spaces. A popular strategy is Bayesian optimization, which aims to find candidates that maximize material properties; however, materials design often requires finding specific subsets of the design space which meet more complex or specialized goals.
Here, a framework is presented that captures experimental goals through straightforward user-defined filtering algorithms. These algorithms are automatically translated into one of three intelligent, parameter-free, sequential data collection strategies (SwitchBAX, InfoBAX, and MeanBAX), bypassing the time-consuming and difficult process of task-specific acquisition function design. This framework is tailored for typical discrete search spaces involving multiple measured physical properties and short time-horizon decision making.
This approach is demonstrated on datasets for TiO2 nanoparticle synthesis and magnetic materials characterization and show that these methods are significantly more efficient than state-of-the-art approaches. This framework provides a practical solution for navigating the complexities of materials design and helps lay groundwork for the accelerated development of advanced materials.